In June 2021, Consumer Reports (CR) released the results of a nationally representative survey related to broadband use. Figure 1 below shows some interesting results from that survey. On the heels of that survey, CR launched its “Let’s Broadband Together” initiative, which uses crowd-sourced methods to gather more data. The most unique aspect of this crowd-sourced data collection effort is that CR asks respondents to upload their broadband bills.
Figure 1: Some Headline Results from CR’s June 2021 Survey
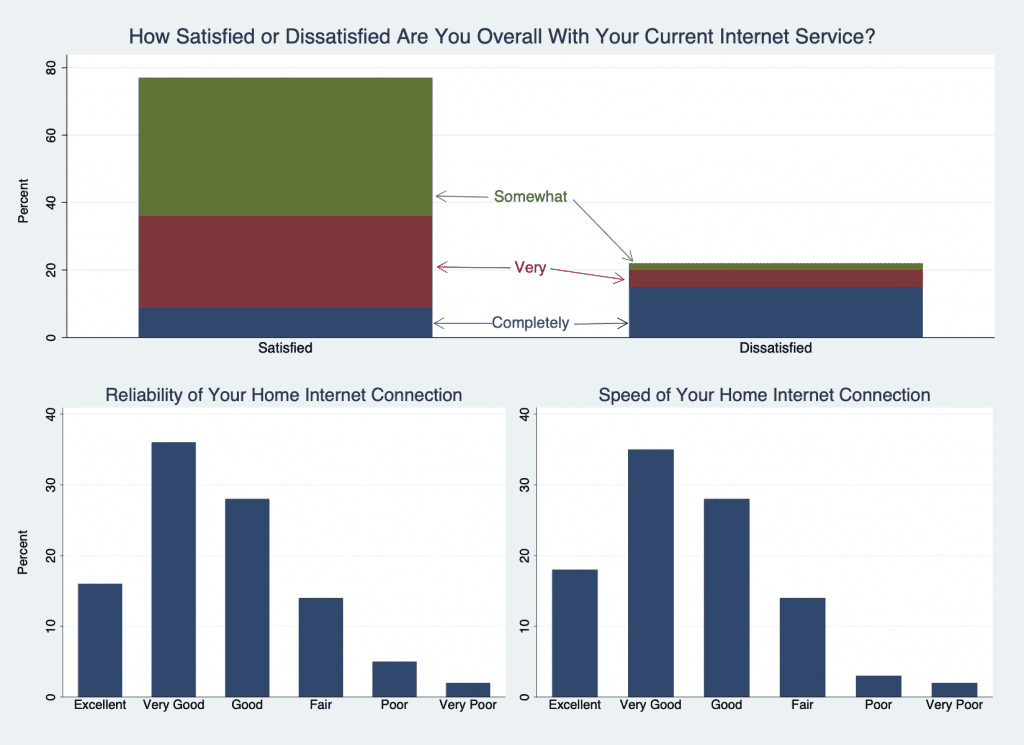
At TPI we love broadband data and analysis. We’ve even built a map that includes many broadband datasets and the tools to analyze them. So as CR collects more broadband data, we want to offer some unsolicited advice on how to make sure its analysis improves our understanding of the broadband marketplace. After all, who doesn’t love unsolicited advice?
We have five suggestions to ensure the data yields its full potential:
- Address the sample selection problem.
- Take advantage of the large sample but avoid p-hacking.
- Describe in detail how prices are derived from the submitted bills.
- Follow M-Lab’s guidelines on how to report its speed results.
- Make the raw data, not just the topline results, publicly available.
Address the Sample-Selection Problem
The most pressing challenge facing analysis of crowd-sourced data is that the data is unlikely to be representative of the population, unlike a well-designed survey that reaches out to people, like the one CR apparently did earlier this year.
Unfortunately, CR may have inadvertently biased its sample by encouraging respondents to participate if they are unhappy with some aspect of their broadband service (see the screenshot below). This survey prompt is likely to bias respondents towards negative sentiments or attract people who hold negative sentiments. Their views are crucial, but may not be representative.
Figure 2: CR “Let’s Broadband Together” Survey Landing Page
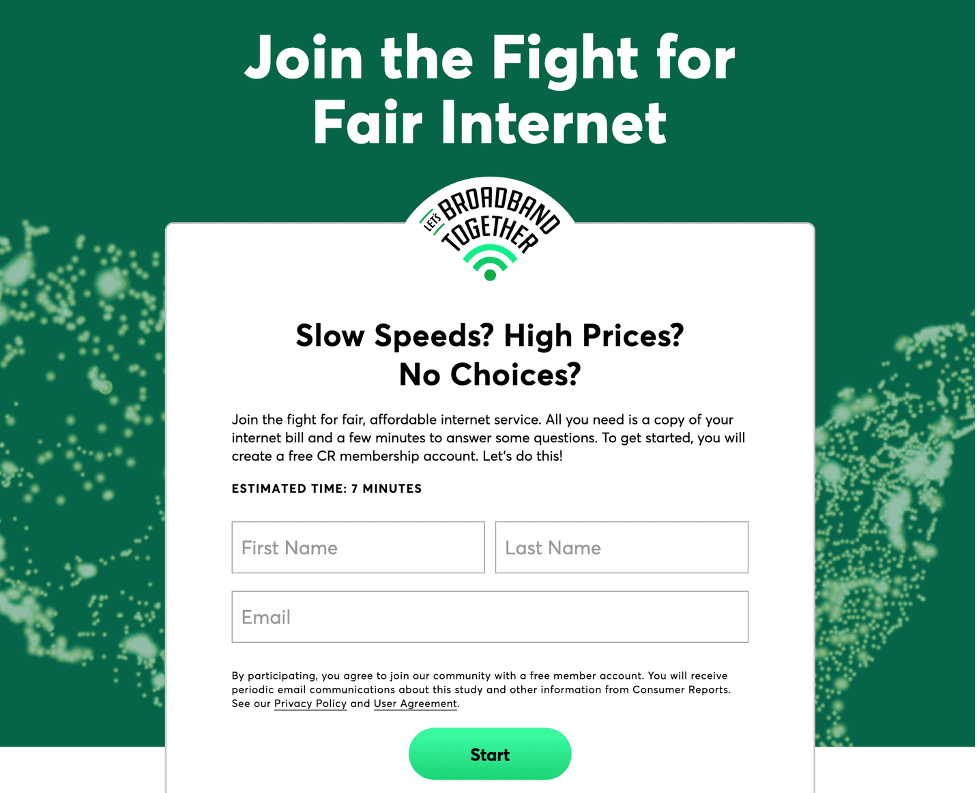
A built-in bias can lead to false conclusions down the road. A famous example is the Literary Digest’s survey of ten million households in 1936, which concluded that Alfred Landon would trounce FDR in the presidential election. A cottage industry of research examining this poll concluded that the error resulted from surveying subscribers and people who owned cars or telephones, which skewed the sample towards wealthier households and contributed to nonresponse bias. Together, those factors meant that people with strong feelings against FDR were more likely to respond to the survey.
Because CR asks its subscribers to reply to the survey, respondents may look more like CR subscribers than the overall population. Respondents don’t have to be subscribers, though, but may be biased in other directions that we don’t know. Are respondents more likely to be wealthy, and therefore possibly less price sensitive, more likely to purchase high end plans, and more likely to be satisfied with their service? Or, contrarily, are respondents more likely to be people who are particularly upset with their broadband and so flock to the survey to vent? Similarly, it seems unlikely that the sample will be geographically representative.
CR should address the selection bias at least by, for example, comparing the demographics of the respondents to means or medians of the actual population. How do the survey respondents compare to the U.S. population in terms of income, gender, race, and education? Are the respondents distributed evenly across the country?
Another possible approach would be for CR to follow up with a random sample of respondents with questions designed to measure the extent of bias. A follow-up survey takes resources—money and time—and may not be feasible.
Take Advantage of the Large Sample but Avoid P-Hacking
As of October 12, 2021, CR reported having more than 50,000 responses to its survey. The relatively large number of responses poses opportunities and pitfalls.
The key opportunities it creates relate to being able to investigate subgroups within the sample. For example, an important new study by Dominique Harrison at the Joint Center discusses broadband problems unique to Black households in the rural U.S. South. With a large enough sample, it may be possible to focus specifically on that group to learn more and, hopefully, envision policy approaches that can lead to improvements. The same point is true for other communities that may face unique issues.
The pitfalls relate to what is known as “p-hacking.” P-hacking refers to fitting the data in various ways until you find a statistically significant result that fits your prior belief even though it does not accurately reflect what the data tell us. Put more colloquially, it involves cutting the data until you find what you are looking for, even though what you have found may have no real meaning.
Probably the best way to ask focused questions about specific communities and to police p-hacking is to release the raw data itself, which is the final recommendation below.
Describe in Detail How Prices are Derived
CR’s approach of looking at actual bills is much better than simply asking people how much they pay for their service. When people are asked how much they pay, they may not have a good framework for teasing out that number, particularly when broadband is bundled with other services, may not respond truthfully, or may not know. Learning what they actually pay by collecting bills should lead to more accurate results.
Having the bill, though, still leaves the problem of how to extract the price of the broadband service itself, particularly in ways that make prices comparable across households. Challenges include:
- How to derive the price for internet service from a price for a bundle that may also include video, home phone, and, increasingly, mobile service;
- How to account for promotional prices;
- How to account for quality differences (e.g. speeds) across plans.
There is no one, best approach to making prices comparable. Any particular approach has advantages and disadvantages and may lead to different normative conclusions about what is “expensive” and “affordable.” For example, one approach to comparing prices is to calculate a price per Mbps, but we need to interpret results carefully. Consider three hypothetical broadband plans. Plan A costs $50 per month for 50 Mbps. Plan B costs $75 per month for 100 Mbps. Plan C costs $300 for a gigabit. The table below shows the normalized price, price per Mbps.
Price | Mbps | Price per Mbps | |
Plan A | $50 | 50 | $1.00 |
Plan B | $75 | 100 | $0.75 |
Plan C | $300 | 1000 | $0.30 |
This normalization shows the gigabit plan being the least expensive. But this approach imposes a value judgment: it assumes that everybody values an additional dollar the same as an additional megabit per second, which might not be true. Some consumers may, indeed, view prices this way, but in earlier research I did with Indiana University’s Jeffrey Prince and Yu-Hsin Liu, we found that people place decreasing value on additional speed as speeds increase. That means some people will prefer the $50 per month plan, even though the price per Mbps is the highest.
Another approach is to group plans by feature. Rather than trying to derive a single price, CR could show prices for standalone broadband, video and broadband bundled together, and other bundles that make sense to group. I did this in a paper with James Riso that examined 25,000 broadband plans across countries. One implication of this approach, however, is that it becomes more difficult to get a sense of how much the internet component of the bundle costs.
Another approach would be to create variables for each aspect of the bill and make those variables available in the raw data. That information would make possible hedonic price analysis that help us learn how different components of a service contract contribute to the final price. It would also make it possible to learn whether certain groups in the population tend to purchase specific services or gravitate to different plans, which might be useful in measuring digital divides.
My point is not to criticize any particular method of calculating comparable prices. Every approach has pros and cons. Instead, the point is to consider carefully the implications of any choices made when calculating price from a bill and provide detailed information on how those calculations were made.
Follow M-Lab’s Guidelines on How to Clearly Report Its Speed Results
Speed test results are subject to debate given that different sites and testing methods measure speeds differently, yielding disparate results. CR’s survey uses M-Labs to test respondents’ speeds. On its site, M-Lab explains how its test works, its limitations, and how it differs from others. Ookla, a large, competing provider of speed tests, explains some differences across tests in a white paper. A recent paper by MIT professor Dave Clark also discusses the M-Labs speed test data.
Given the many approaches to testing speeds, M-Labs itself has laid out guidelines on how to represent its speed data to avoid misinterpretation. When presenting its speed test results, CR should take care to follow those guidelines.
Make the Raw Data, Not Just the Topline Results, Publicly Available
Yes, this piece of advice is self-serving, because if CR’s data is public we will add it to the collection of data on our TPI Broadband Map.
Self-serving or not, the raw data is far more valuable than the topline results for many reasons.
First, topline results omit interesting and potentially important within- or across-group results and allow for important controls that might help assign causality—and therefore point to solutions—rather than simple correlations that may or may not be meaningful. With the raw data, researchers may be able to correct for some of that problem. After all, just last week the Nobel Prize for Economics was awarded to economists responsible for methods designed to separate correlation and causation from observational data.
Second, allowing researchers access to the raw data will allow for insights that the topline results alone and a report by one group may not find. A lot of people working on a problem is almost always more likely to lead to new insights than a few people.
Third, any dataset is more valuable when it can be combined with other datasets. Making the raw data available should make it possible to combine with data from the FCC, the American Community Survey, and more.
Releasing raw data is increasingly recognized as an important part of presenting original research results. Refereed academic journals now often require authors to make their data available. For example, “It is the policy of the American Economic Association to publish papers only if the data and code used in the analysis are clearly and precisely documented and access to the data and code is non-exclusive to the authors.”
CR cannot, of course, release the data as-is. It must be anonymized, which means removing any personal identifiable information (PII). But removing PII from household broadband bills and showing geographic location at, say, the zip code or county level, should make it impossible to identify respondents. There is significant precedent for releasing anonymized person- or household-level data this way, including the U.S. Census Public Use Microdata Sample for the American Community Survey, and the CDC’s National Health Interview Survey.
Anyone with an agenda could, of course, abuse the raw data, be it to make U.S. broadband look especially good or especially bad. Hopefully, though, the availability of the raw data will also help to police and prevent this kind of misuse of statistics.
Conclusion
CR’s survey data can be an important input to the national search for data that can help inform how we allocate broadband resources. To best inform the national debate and get the most value out of this data, CR should address the sample selection issues, use the large sample to investigate subgroups of the population while avoiding the temptations of p-hacking, explain in detail how it derives prices from the bills it collects, follow presentation guidelines from the provider of the speed test it is using, and make the raw data available for researchers to explore.
Collecting data is only half of the journey toward evidence-based policymaking. Analysis matters, too. Hopefully CR will endeavor to help the country make the best use of its data.